The Power of Data Labeling in Machine Learning
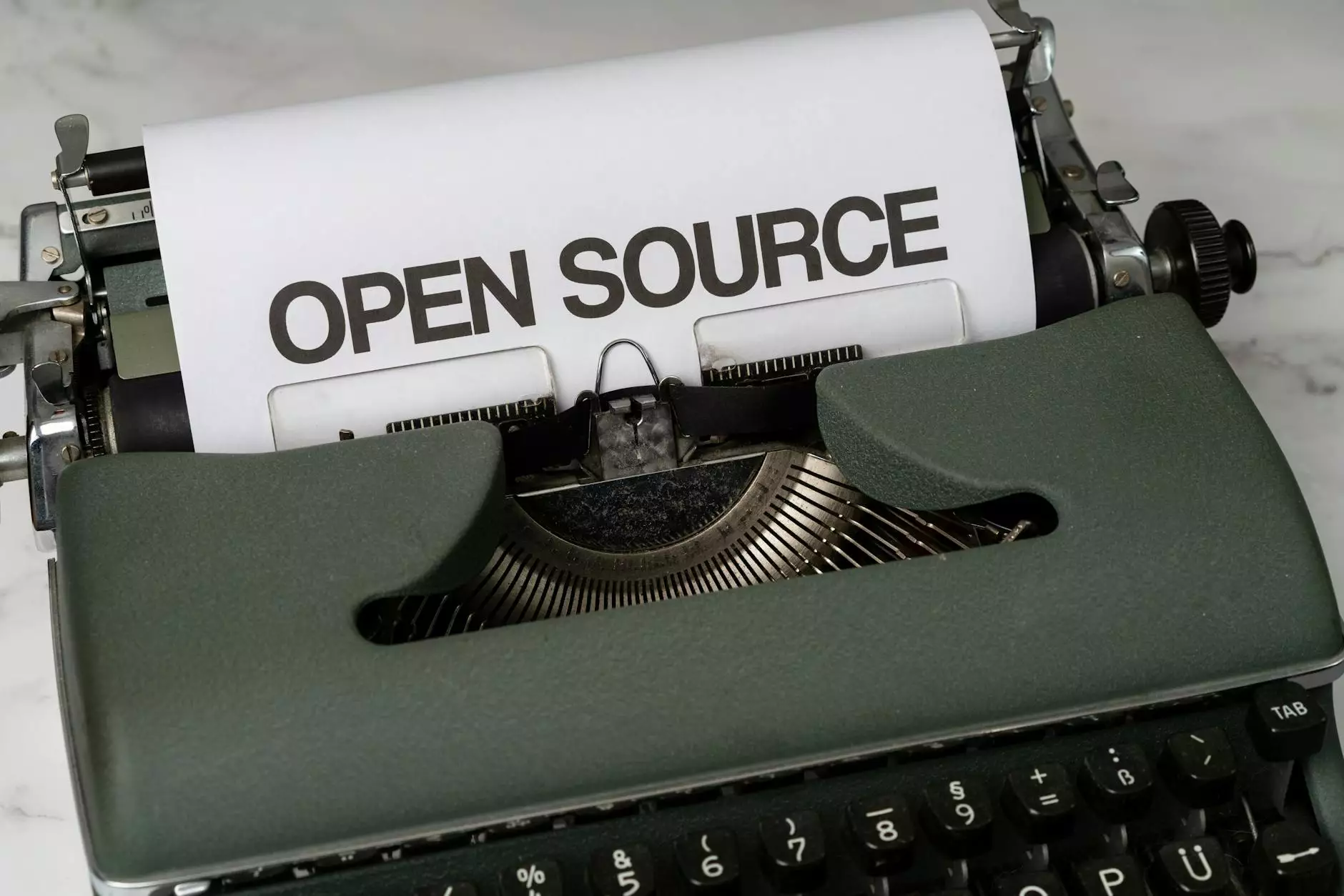
In the rapidly evolving world of machine learning, one key aspect that significantly impacts the performance and accuracy of models is data labeling. At Keylabs.ai, a leading provider of IT Services & Computer Repair, we understand the critical role that data labeling plays in the success of machine learning projects.
Understanding Data Labeling
Data labeling involves the process of annotating or tagging data to provide context and meaning to raw information. This labeled data serves as the training material for machine learning algorithms, enabling them to learn and make accurate predictions.
Whether it's identifying objects in images, transcribing audio recordings, or categorizing text data, data labeling is essential for supervised learning tasks. It helps machines recognize patterns and make informed decisions based on the labeled examples provided.
The Importance of Quality Data Labeling
High-quality data labeling is crucial for the success of machine learning models. The accuracy and relevance of the annotations directly impact the performance and generalization abilities of the algorithms. At Keylabs.ai, we emphasize the importance of precision and consistency in data labeling to ensure reliable results.
By meticulously labeling training data, we help our clients build robust machine learning models that can effectively address complex problems across various industries. Our expertise in data labeling sets us apart as a trusted partner in the ever-changing landscape of artificial intelligence.
Benefits of Effective Data Labeling
Effective data labeling offers a multitude of benefits for machine learning projects:
- Improved Model Accuracy: Well-labeled data leads to more accurate model predictions and classifications.
- Enhanced Data Quality: Proper labeling ensures the cleanliness and relevancy of the training dataset.
- Increased Efficiency: Well-organized data labeling processes optimize the training time and resource utilization.
- Greater Predictive Power: Quality annotations enable models to make better predictions on unseen data.
Data Labeling Techniques
There are several techniques used in data labeling to handle different types of input data:
- Bounding Box Labeling: Commonly used for object detection tasks, bounding box labeling defines the location and size of objects within an image.
- Pixel-Wise Segmentation: Ideal for image segmentation, this technique assigns labels to individual pixels to outline different objects in an image.
- Text Classification: For natural language processing tasks, text classification involves labeling text data with predefined categories or tags.
Conclusion
As businesses continue to harness the power of machine learning to drive innovation and growth, the role of data labeling becomes increasingly vital. At Keylabs.ai, we recognize the significance of accurate and reliable data labeling in building advanced machine learning solutions that deliver tangible results.
By partnering with us, you can leverage our expertise in IT Services & Computer Repair to elevate your machine learning projects and stay ahead in today's competitive landscape. Join us in unlocking the full potential of data labeling in machine learning and propel your business towards success.
data labeling machine learning